1. Dose Adjustments as Personalized Neuromodulation
Neuromodulation dose is defined as those aspects of the technology that impact how energy is applied to the body (PMID: 22305345). These are:
1) Amplitude of the stimulation, which is the peak intensity, whether voltage-controlled (expressed in volts) or current-controlled (expressed in amperes); 2) Location where energy enters the body. Location refers here only to the relevant interface between the device and body (not all device parts). For electrical stimulation location is the size and placement of electrodes; 3) Waveform / timing of the stimulation, which spans how long therapy is applied, the interval between treatments, and how intensity changes during treatment (such as frequency and pulse duration).
Neuromodulation dose and the properties of the body together determine which cells are exposed to what energy, which in turn drives the outcomes of neuromodulation. In this sense, two devices that provide an identical dose are indistinguishable to the body – they are same in the therapeutic responses they produce. Device parameters outside of dose, such as its user interface and battery life, certainly matter, but are not part of the therapeutic dose. Therefore, when we personalize neuromodulation dose we adjust only: amplitude, location, or waveform/timing.
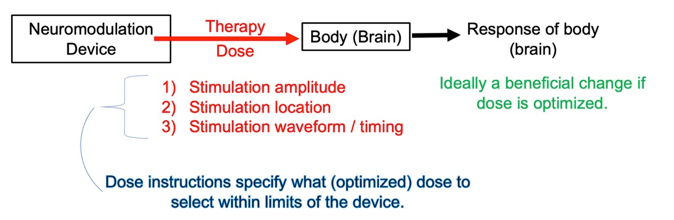
Figure: Neuromodulation dose can be adjusted by amplitude, location, or waveform / timing. A single neuromodulation device may provide a range of possible doses. Dose instructions indicate how to adjust (optimize) dose to produce desired benefits.
Each neuromodulation device is designed to provide a limited range (set) of neuromodulation doses. Each neuromodulation devices limits its range of dose parameters based on technology (e.g., hardware limitations), the use-case (intended use), and safety standards (PMID: 15661300; PMID: 33243615; PMID: 37201865). This does not mean it is advisable to try any dose available from a device; for example, some doses may be painful. A single neuromodulation device may allow a few or thousands of possible doses – that is, combinations of amplitude, location and waveform / timing. Given too many dose possibilities to practically test and a cost to testing ineffective doses (lost time, side-effects), there must be a therapy technique to search for and select a preferred dose; this process is neuromodulation dose instructions.
Individualized therapy with drugs is limited to gross adjustment of amount (e.g., number of pills) or timing (e.g., twice per day). Small changes in drug molecular formulation can be lethal. With pharmacotherapy, there a way to change formulation “on the fly” (after production and distribution). Neuromodulation devices are designed to allow “on the fly” adjustment of dose. This allows therapy to personalized, even on a treatment-by-treatment basis. Dose can be adjusted mid-treatment because there is an instant corresponding change in energy in the body. The kinetics of drug delivery are comparably sluggish.
By adjusting device location, neuromodulation can deliver energy to a targeted brain region or several brain regions, in contrast to systematically distributed drugs. Changing device location allows engaging distinct brain regions that are implicated in disease etiology or its control. A device location may be changed by moving electrodes, for example adjusting a TENS device over a painful body region. In situations where a device has several electrodes already placed, for example an implanted lead with 4 electrodes, device location may be changed by selecting which electrodes are activated.
Thus, neuromodulation devices are adjustable “on the fly” and with specificity in both time and brain regions. When dose is adjusted for each individual, it is personalized. In order to select for each individual among many possible doses, dose instructions rely on biomarkers from individuals. I describe how biomarkers are used in dose instructions – in doing so I identify distinct classes of biomarkers in neuromodulation.
2. The When and Why of Biomarkers in Neuromodulation
In searching for example of how biomarkers are used to personalize neuromodulation dose, one struggles to find examples where this is not the case. The use of biomarkers in neuromodulation is so ubiquitous it can go unrecognized. I believe every FDA-approved neuromodulation is personalized based on biomarkers. Transcranial Magnetic Stimulation (TMS) dose is adjusted based on an observable finger twitch. Electroconvulsive Therapy (ECT) dose is adjusted until a seizure is produced. Many treatments for pain, such a Transcutaneous Electrical Nerve Stimulation (TENS) or Spinal Cord Stimulation (SCS) are conventionally adjusted so that a tingling (paresthesia) is felt by the patient over the painful region.
While these biomarkers are used to adjust dose, biomarkers are not the goals of the treatment, which are rather the clinical endpoints. Biomarkers are thus a means to an end. Moreover, some biomarkers seem peculiar relative to the associated clinical syndrome. What does a finger twitch by TMS tell us about treating suicidal ideation? Why would feeling mild electrical zaps during TENS or SCS reduce chronic pain?
This ubiquity of biomarkers in neuromodulation leads to two related questions:
1) How are biomarkers used to personalize neuromodulation, as they are not (and often not even clearly related to) clinical endpoints?
2) Why does neuromodulation (as opposed to drug therapies) seemingly necessitate personalized interventions using biomarkers?
The first question is a description of therapy techniques and specifically of dose instructions. My thesis is that a clear analysis of techniques requires distinguishing different types of biomarkers. The second question cannot be answered with first explaining the techniques of neuromodulation personalization.
I define a biomarker as
any measurement taken from an individual or observation made by the patient or care-giver that is used to adjust (or withhold) neuromodulation dose, and that is not a clinical endpoint
For example, a biomarker may determine if no therapy should be given, or a low treatment dose, or a high treatment dose. I include subject self-reports as biomarkers (while in other contexts biomarkers might be limited to objective measures). This is because I explain biomarkers by how they are used.
Two general classes of biomarkers are distinguished:
Responsive biomarkers are used to adjust treatment based on their change in response to treatment, so measured after treatment starts.
Predictive biomarkers are used to adjust treatment without (or irrespective of) changing with treatment. They can be measured before or after treatment starts.
A predictive biomarker may be discovered to change with the intervention and so be re-considered a responsive biomarker (and vice versa). But my analysis of therapy technique is based on how biomarkers are used, specifically what biomarker type they are assumed to be for dose instructions.
A responsive biomarker is measured after the therapy starts, during or after stimulation. Based on the responsive biomarker, a decision regarding how to update therapy is made. This can be repeated: a first treatment dose, a first measured biomarker, second treatment dose, and second measured biomarker, and so on. This treatment technique is a loop. In each turn around the loop, the treatment dose is (hopefully) adjusted toward a more useful one. As this process is iterative and goal directed, we can say dose is tuned.
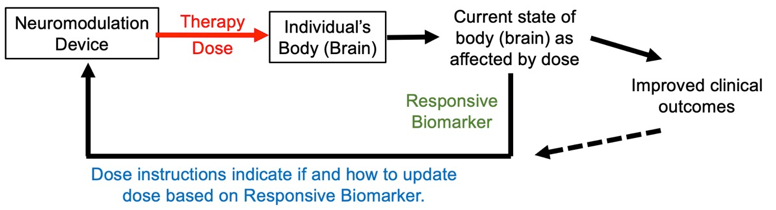
Figure: Basic loop of personalized neuromodulation using responsive biomarker.
The usefulness of a responsive biomarker depends on achieving better clinical outcomes when patients receiving individually tuned doses versus the same fixed treatment dose. This requires anticipating how clinical outcomes will change based on the responsive biomarker.
A predictive biomarker can be measured and a treatment plan made before initiation of therapy, or it may be measured after therapy starts to update the course of treatment. The usefulness of a predictive biomarker depends on clinical outcomes being better with patients segregated into distinct treatment doses (or no dose) versus all patients receiving the same treatment dose. This requires anticipating how clinical outcomes will change with treatments based on the predictive biomarker. While a predictive biomarker is not expected to change as a result of therapy dose, it may change over time for other reasons, and so may be measured repeatedly – for example measured before each treatment session. If measured more than once, the predictive biomarker is use in a loop.
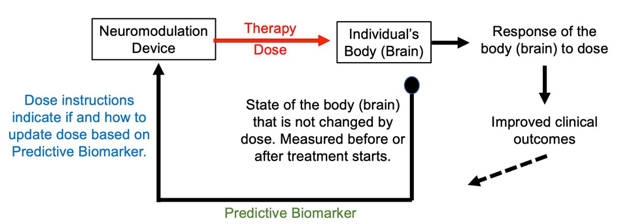
Figure: Basic loop of personalized neuromodulation using predictive biomarker.
My central thesis is the assumption about the nature of the biomarker determines how the biomarker is used in personalizing treatment. In the next sections I will explain subtypes of responsive biomarkers and of predictive biomarkers, and how their distinction informs treatment techniques. These biomarker-specific treatment techniques are distinct treatment loops
Why not measure how clinical outcomes respond to treatment and use that to update treatments? This is certainly done (dashed line above). The usefulness of biomarkers depends on how they improve treatment dose optimization compared to relying only on clinical endpoints.
Each neuromodulation device offers many dose options. Dose instructions are the rules by which a candidate treatment dose is selected and, as needed, updated. Dose can be tuned to each patient in principle, but to do so in practice requires a method to select and screen multiple candidate dose - that is for sufficient iterations in dose tuning (i.e., times around the loop). If we rely only on clinical endpoints and if clinical changes are slow (e.g., months) this would severely limit tuning (e.g., one new dose/loop every few months). Such delays are especially problematic with patients desperate for benefits, when poorly selected doses aggravate side-effects, and/or when there are few good doses among many possible dose options. Biomarkers are the dose-search accelerant: a measurement which (in the context of its associated dose instructions) anticipates changes in clinical endpoints by a given dose.
For responsive biomarkers to anticipate clinical changes they should 1) change faster or be easier to measure than the clinical endpoints and 2) change in a manner that guides updating dose (based on dose instructions) to improve the clinical endpoints. For predictive markers to anticipate clinical changes, they should inform the updating of candidate doses (in a process specified by dose instructions) for improving clinical endpoints more effectivity than relying only on clinical endpoints. We cannot disassociate the usefulness of any biomarker from its associated dose instructions - which we shall see is specified in the structure of loops. And conversely, we are reminded that dose instructions are the process of selecting a dose based on the assumed significance of the biomarker.
For all the diversity of biomarkers and dose instructions, dose adjustment can only be of amplitude, position, or waveform/timing, and as limited by the device and use-case. For the most part, the biomarker types and associated loop structures developed here are agnostic to which aspects of dose are adjusted, and are universal to all neuromodulation devices. What follows is a categorization of biomarker sub-types based on how they are used in therapy technique (loops). My explanation focuses on using biomarkers as guides toward a desired clinical outcome, though they could be used to avoid side-effects. Many neuromodulation dose instructions are intended to find a ‘therapeutic window’: a dose with sufficient to provide efficacy but insufficient to produce (unacceptable) side-effects. A given biomarker may be used specifically for efficacy or for side-effects. Or the same biomarker may be used to tune dose to provide efficacy and also minimize side-effects.
My explanation is based on clinical endpoints and so treatments, but this analysis of biomarker types and their associated loops apply equally to performance enhancement endpoints (in healthy individuals).
My explanation develops concepts at schematic level (simple block diagram) which is the appropriate level of description for the underling theory. The scheme is the biomarker-specific dose instructions, which generally take the form a loop starting with the biomarker readouts and ending with an updated doses.
3. Clinical Endpoints and Three Types of Responsive Biomarkers
Clinical endpoints are the ultimate medical or performance objectives of the intervention. Clinical endpoints are what we, especially the patient, hope to achieve. Such as the reduction of tremor, relief of pain, or improvement of mood. There are established rating scales (including those based on subjective reports) that are accepted as a basis for disease diagnosis and burden, and hence for measuring improvement with treatment. Clinical endpoints are used in clinical trials and regulatory submissions (e.g., evidence-based medicine), where the reliance of biomarkers in lieu of clinical endpoints can be controversial. Patients may have additional goals related to quality of life (e.g., ability to pick up their child, return to work) that can factor (informally) into clinical endpoints.
The first type of responsive biomarker is defined as a surrogate to a clinical endpoint. The essential idea is that this biomarker changes alongside clinical symptoms, when one gets better so does the other. Hence, a therapy dose that changes a biomarker of the first type in a preferred direction, will also improve the clinical endpoint.
For some neurological diseases, instruments that measure brain function (EEG, MRI) can provide objective biomarkers of disease progression and serve as surrogates to the clinical endpoint. For example, abnormal brain electrical activity (biomarker) indicating risk of seizures (seizure control is the clinical endpoint in epilepsy treatment). For many diseases, such as pain and depression, there are no generally established objective biomarkers of the first kind (there is no instrument to measure “suffering”); at least so far as can be used (with sufficient sensitivity and specificity) as a type one biomarker (clinical surrogate) for dose optimization.
A subjective or objective measure of brain activity may be used as the clinical endpoint in one use-case, but a type 1 biomarker in another. This can be an arbitrary distinction, governed by external conventions (e.g., what a medical society or the FDA accepts for the scoring of a disease severity). Thus, depending on context, a patient’s report of improved symptoms is a clinical-endpoint (e.g., part of a validated clinical scale) or a subjective biomarker of a first type (indicative of therapy working but not itself the primary goal of therapy).
Failure to explicitly distinguish between clinical endpoint and biomarkers of the first type is problematic for several reasons. It may be discovered (for some patients) that the biomarker does not track the clinical endpoint in a meaningful way. Distinguishing between first type biomarker and clinical endpoints allows for the former to be selected (and dose instructions applied) specifically to accelerate dose tuning to improve the latter.
For responsive biomarkers of the first type to be useful in tuning neuromodulation devices, they should respond to a well-selected dose in a manner faster or easier to resolve than the clinical endpoint. Otherwise, one can simply use the clinical end-point. Faster to resolve means the biomarkers changes in response to neuromodulation before a detectable change in the clinical endpoint. This can be invaluable if the clinical endpoint takes weeks or months to change, but the biomarker responds rapidly. Easier to resolve can mean an objective biomarker as opposed to a subjective clinical endpoint. Easier may also mean that the tool used to access the biomarker of the first type is cheaper or less burdensome to collect - for example self-administered internet questionnaire versus in-person assessment by a clinician; or a home heart-rate monitor versus an in-clinic EKG. I use the word anticipate to indicate some combination of more rapid or simple biomarker measurement compared to the clinical endpoint.
The definition here used for a type 1 biomarker in neuromodulation, largely follows the NIH-FDA BEST guidance “Surrogate endpoint biomarker: A response biomarker that is an endpoint used in clinical trials as a substitute for a direct measure of how a patient feels, functions, or survives. A surrogate endpoint does not measure the clinical benefit of primary interest in and of itself, but rather is expected to predict that clinical benefit or harm.”
To summarize definitions of clinical endpoints and biomarkers of the first type in neuromodulation:
Clinical endpoints are the primary goals of the intervention, whether defined by the care-giver, patient, and/or regulator. They are the outcomes the device will be primarily marketed or prescribed for. For effective therapy, clinical endpoints improve with well selected dose.
Responsive biomarkers of the first type change with a stimulation dose corresponding to, and ideally anticipating, changes in a clinical endpoint. So far as a clinical endpoint are durable (slow changing) so must be associated biomarkers of the first.
Based on the distinctions above, that a measure that is a clinical endpoint in one use-case may be a responsive biomarker of the first type in another use-case. Biomarkers are not clinical endpoints but a means to an end.
A biomarker of the first kind does not need to perfectly track or predict changes in clinical endpoints in every patient in order to still be useful. Biomarkers of the first kind, that are both fast and reliable, are rare in brain disorders.
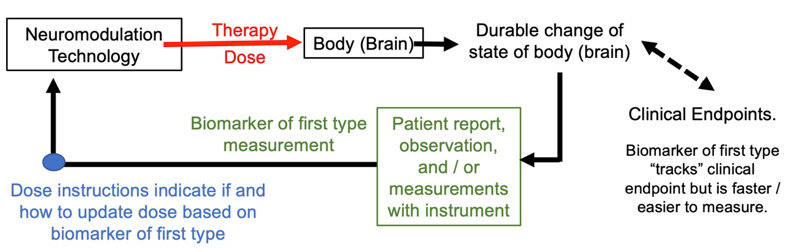
Figure: Basic dose tuning loop using biomarker of first kind in dose optimization loops. Biomarker of the first type and clinical endpoints are sufficiently correlated so that changes in the former can herald improvements in the latter
The second type of biomarker can be thought of as the measurement of the theory of mechanism of action. This second kind of biomarker is sometimes called a measure of “target engagement”. Target engagement shows you are activating (targeting) the biological process; you assume is driving the therapy outcome. We thus assume we are measuring not just any random body response to stimulation, but the response (process) that then mediates the therapy (the improvement in clinician endpoint). If we can adjust the stimulation dose until this biomarker of the second type (measure of target engagement) moves in the preferred direction, this means that the symptoms should (eventually) also improve. And so, the second kind of biomarker is a signal we can use to tune the dose.
The use of the biomarker of the second type in dose optimization loop is shown in the figure below. Note the general approach shown in the figure looks similar to the first type of biomarker, except an emphasis on short term changes / mechanism. This is a distinction with a difference in how dose tuning is practiced.
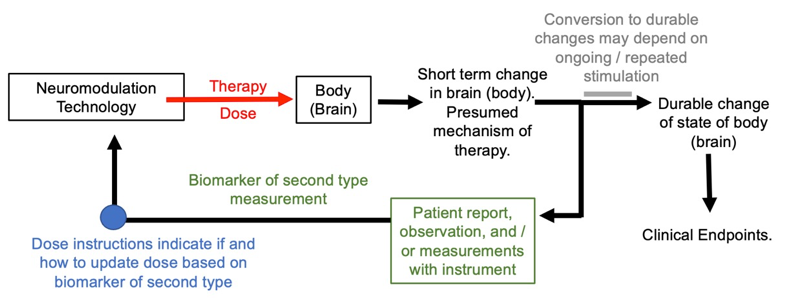
Figure: Basic dose tuning loop using biomarker of second type. The biomarker of the second time is assumed to track a mechanism of therapy.
Consider TENS and SCS for pain. TENS and SCS for pain generally adjust dose to produce tingling (paresthesia) of the body region with pain. The tingling is presumed to be the second kind of biomarker: showing that the therapeutic pathway has be activated. Specifically, this is based on the mechanism theory of gate control, whereby the tingling sensation masks the pain (much like rubbing a body part when its injured). This method of dose adjustment is intended to prevent both under-dosing leading to no therapy (e.g., no tingling with too low amplitude) and over-dosing leading to side effects (e.g., discomfort with too high amplitude). In pain control, tingling is presumed to be a biomarker of the second kind as it indicates a dose that should be therapeutically effective while not, in itself, directly associated with chronic pain. Namely, producing tingling with stimulation does not, one way or another, measure the clinical endpoint of chronic pain. One can produce the same tingling in a person with no chronic pain.
Like the biomarker of the first type, usefulness of biomarkers of the second type in therapy optimization depends on the biomarker being faster to change or easier to measure than the clinical endpoint. Actually, this anticipation is expected from a biomarker of the second type: if we are stimulating the process presumed to lead to improvement in symptoms, surely that process will change before symptoms change. But the reason for a fast response goes further. Unlike the biomarker of the first type, biomarkers of the second type may respond acutely to stimulation and then return to their initial state.
As an analogy, consider someone using a heating pad for a sore joint. Measuring the increasing temperature of the joint during therapy is a biomarker of the second type – one expects tissue heating dependent mechanisms to drive therapy outcome. But after a heating pad therapy, as the joint feels better, we do not expect body temperature to be chronically raised – temperature is not a biomarker of the first kind.
What about tingling in treatment of pain? If the relief of pain continues after stimulation is stopped (and tingling stops) will depend on if there are durable changes. Different approaches are possible. For implanted devices, stimulation can be ongoing, so that enduring effects are not required, though may occur. For non-invasive devices, which practically cannot be worn all the time, pain relief may be either acute, especially if applied in response to a painful event (e.g., tissue strain, headache), or pain relief continue as a result of enduring change outlasting stimulation. Even if pain relief is not enduring, the tingling producing by stimulation is not considered a surrogate of pain.
What if a biomarker acts as both a surrogate to the clinical outcome and also represents a presumed mechanism of action? For example, there is a pathological brain process that is being directly fixed by stimulation (the mechanism of action) where that brain process it also directly related to clinical endpoints (its enduring changes correlate with symptoms). Conversely, can one argue that any biomarker that improves alongside with clinical symptoms. and so, as a surrogate biomarker, might be a mechanism of action – especially if the biomarker is objective and changes before (anticipates) the clinical symptoms? What if clinical endpoints are improved only during stimulation, so that a surrogate biomarker is also not enduring (after stimulation stops)? All these options are reasonable and, in some applications, expected. The biomarker classification here remains clear and logically consistent, so long as a hierarchy is defined:
A biomarker of the second type measures a presumed therapeutic mechanistic process and is not a type one biomarker. The type two biomarker is a conjectured mechanism of action. Its engagement is considered essential for therapeutic outcomes.
A biomarker of the first type is a clinical surrogate in so far as it tracks (or anticipates) clinical endpoints. When clinical endpoints are durable, so is the biomarker of the first type. A biomarker of the first type may or may not also be considered a mechanism of action.
From the above we see that any biomarker whose changes are as slow (durable) as the clinical endpoint is a type one biomarker, irrespective of it is assumed to be a mechanism of action. Indeed, ideas about mechanisms of action may change. This distinction is consistent with my approach to distinguish biomarkers by how they are practically used in therapeutic techniques.
The third type of responsive biomarker is electrodynamics. Neuromodulation dose in combination with anatomical and tissue properties governs the energy profile generated in the body (PMID: 22305345). For electrical and magnetic stimulation, that energy is electrical current flow generated in the body, expressed as regional electric field or current density. Because anatomy and tissue properties vary across individuals, there is not a generic correspondence between dose and the energy cells are exposed to. The outcomes of stimulation are determined by this energy profile (e.g., the electric field distribution in the nervous system). This can be measured, for example using a volt-meter to measure the electric field. Any electromagnetic energy produced is generated instantly when the device output is activated, and dissipates instantly when the device output is deactivated. The measurement of energy may than be used as a responsive biomarker, as shown in the figure below.
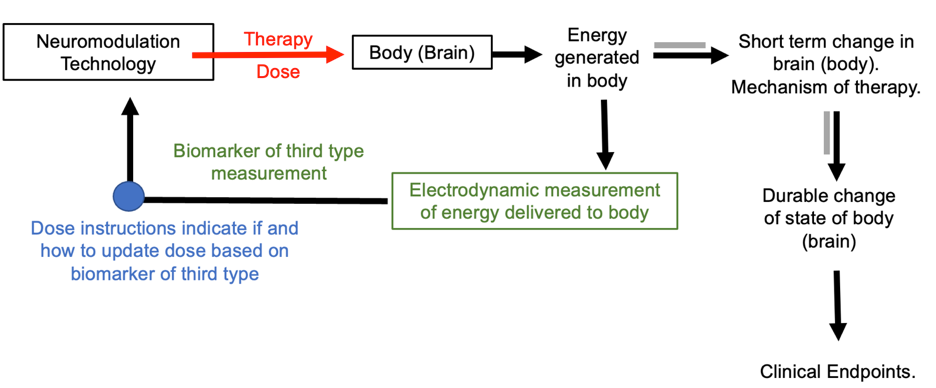
Figure: Basic dose tuning loop using responsive biomarker of third type. The biomarker of the third time measures energy generated in (or around) the body.
Measurement of resistance or impedance, which is ubiquitous in electrical stimulation devices, can be a rudimentary biomarker of the third type. Such measurements are typically used to detect a device fault (in which case not as a biomarker) or the tissue resistivity around electrodes (which is an electrodynamic biomarker). Impedance measures may be used to stop or adjust stimulation location (away from a high impedance area), and so are part of dose instructions.
A type 3 responsive biomarker is an objective measurement of the energy applied to the body, that is present only when the stimulation is active. Its relation to stimulation dose is governed by physics and anatomical / tissue properties. A type 3 biomarker is not a mechanism of action or clinical surrogate. In addition to initiating instantly with device output, type 3 biomarkers are as stable as device output.
A type 2 responsive biomarker is a subjective or objective measures, that is a presumed mechanism of action. The mechanism is activated by the presence of this energy, so one would expect to see changes in biomarker type 1 in body (brain) regions exposed to significant energy, or connected to those regions. What mechanisms is activated depends on the energy location and type. The time-course of type 2 biomarkers can be slower (and cumulative with time) than the energy applied. The mechanisms may persist but only acutely (briefly) after the energy / device is deactivated.
A type 1 responsive biomarker is a measurable subjective or objective change that tracks, so is as durable, as the clinical endpoints.
Each responsive biomarker type is thus distinguished by its (presumed) time course.
While the effects on the body are governed by the generated energy, simply knowing the energy generated does not establish (strictly determine) what the outcomes will be. Those outcomes will vary across individuals (depending for example on their brain state), are multi-faceted, and complex. Using biomarkers of the third type to adjust neuromodulation dose is useful in so far as tissue energy level can be linked to clinical endpoints. Hence energy levels can be adjusted to tune dose for optimized clinical outcomes. Biomarkers of the third type are somewhat analogous in drugs to a pharmacodynamic biomarker that measures drug (or drug byproduct) concentration at a target “without necessarily drawing conclusions about efficacy or disease outcome or necessarily linking this activity to an established mechanism of action” (FDA-NIH BEST Resource).
4. Predictive Biomarkers including Play-back, Neuronavigation, Gated, and Evoked
There is a broad swath of predictive biomarkers used in medicine to inform if a patient is a candidate for treatment or to stratify patients to distinct treatment doses. Some biomarkers used in neuromodulation treatment planning are similar to those considered in drug therapy. They align with the FDA-NIH BEST definition of “A predictive biomarker is used to identify individuals who are more likely to respond to exposure to a particular medical product...The response could be a symptomatic benefit, improved survival, or an adverse effect.” Here I address the use of predictive biomarkers of special relevance and peculiar implementation in neuromodulation. Whereas each type of responsive biomarker was defined to be exclusive of the others, a predictive biomarker may be multiple of the types explained below.
Maintaining a focus on treatment technique, I consider how predictive biomarker type impact distinct dose optimization loops. Predictive biomarkers can be used to personalize neuromodulation: 1) Prior to treatment, in treatment planning; 2) Immediately before treatment during treatment installation or set-up; or 3) during treatment (online). As always, neuromodulation dose adjustment is only through amplitude, location, and waveform/timing; and dose-instructions explain how to update dose based on biomarkers.
All therapy, including neuromodulation, is governed by inclusion and exclusion criteria - individual traits that nominally allow or exclude, respectively, patients from treatment. These are factors considered before a patient is enrolled for treatment. Inclusion / exclusion rules conventionally do not guide dose tuning. Some inclusion/exclusion may be based on absence of information – for example pregnancy may be an exclusion for a treatment only because pregnant individuals were excluded from prior tests. Or they may be based on non-medical consideration such as cost of treatment or a clinical trial (PMID: 25332910). When an inclusion/exclusion rule is directly shown to predict patient response to treatment (if or how effective at least one neuromodulation dose will be), it is a predictive biomarker.
An inclusion factor may serve a predictive biomarker and as a clinical endpoint; the same assessment conducted before and at the end of treatment. A biomarker may use as predictive in one stage of treatment (where it does not change) and a responsive biomarker in another stage of treatment (where it responds to therapy dose). Biomarkers classification here are based on treatment technique and dual use in distinct treatment stages is not problematic. There will be associated relevant dose instructions for each stage.
Brain Computer Interfaces (BCI) are devices that record and interpret signal from the brain for the purpose of controlling a device, such as a cursor on a screen or a (robot) motor. BCI is therefore intended to measure a subject’s “intent” (or at least a signal that the subject can produce with the intent to control a device). Some BCI uses stimulation either 1) as part of diagnosing brain state (input); or 2) as the function of the device (output)– specifically to stimulate a body part to produce an action. For example, an individual with a spinal cord injury may think about moving a limb, that intent is recorded by a BCI device, and then the device uses electrical stimulation to move the limb. The generation of motor (or sensory) responses by neuromodulation can be called Functional Electrical Stimulation (FES). So, BCI can be used to provide the “intent” to activate FES. This may be called a predictive intent biomarker. The response of the target muscle (or nerve) can also be recorded to updated FES dose; this is then a responsive biomarker. Both the predictive intent biomarker and the responsive motion biomarker will be considered through dose instructions to determine how to update dose.
Play-backis a dose instruction technique with a predictive biomarker. Essentially a therapy dose is considered optimized if it “mimics” one or more aspects of the targeted brain activity. For example, brain activity may be recorded from a specific brain region, and stimulation directed back (by adjusting location) to the same brain region. Spatial playback can be automated using the principles of “reciprocity” (PMID: 28578130). Or the waveform of brain activity may be measured (especially brain oscillation frequency) and the same waveform then used for stimulation (for example a stimulation frequency matching the endogenous activity frequency; PMID: 24167483).
As show below, the play-back biomarker measurement may be made once in treatment planning if it is assumed to be relatively stable, or measured repeatedly (during treatment) if it is assumed to change. However, play-back using predictive biomarkers always assumes the biomarkers do not change with stimulation; or rather, the treatment technique and dose instructions proceed agnostic to if stimulation changes this biomarker.
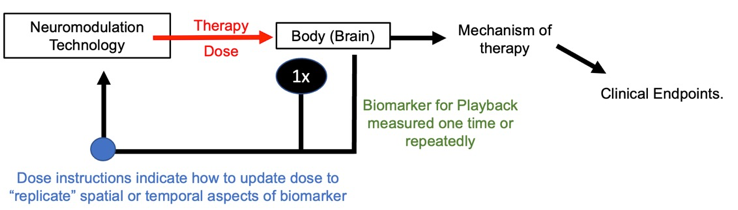
Figure: Dose instructions for predictive playback biomarker.
A specific example of the playback biomarker marker is measuring brain activity using EEG to decide both the position and frequency of high-definition transcranial alternating current stimulation (HD-tACS) (PMID: 35995877). Here one needs to be nuanced: there may be distinct examples where HD-tACS is intended to change the EEG activity (oscillations for example) and this outcome is monitored as part of dose instructions - at which point this is no longer a predictive responsive marker but rather a responsive biomarker of the second type. One can ask: what if we do not know if the oscillations change but, in any case, do not measure them. The biomarker system developed here is agnostic to mechanisms and rather specific to treatment technique, and so this is predictive biomarker.
A neuronavigation predictive biomarker is a measure (scan) of body anatomy combined with a measure of device location used to adjust location. Examples of neuronavigation including fluoroscopy for guiding SCS lead position, interventional MRI-Guided Deep Brain Stimulation lead implantation, and TMS position guided by patient head scans – in all cases using individual anatomy to determine device location. In none of these cases is the anatomy changed by neuromodulation – evidently, the biomarker is predictive, not responsive.
The position of the device is measured repeatedly, so in a loop, in order to iteratively adjust the device location appropriately with the body (shown below). The dose instructions explain how to adjust the device location. Special equipment used for this process are neuronavigation devices. The neuronavigation may be used in the set-up / installation stage (with no device output), and the therapy dose is provided in a second stage. Or neuronavigation may be used to maintain position during treatment. Also shown below (dashed line) is that additional biomarkers and loops may be implemented alongside neuronavigation predictive biomarkers.
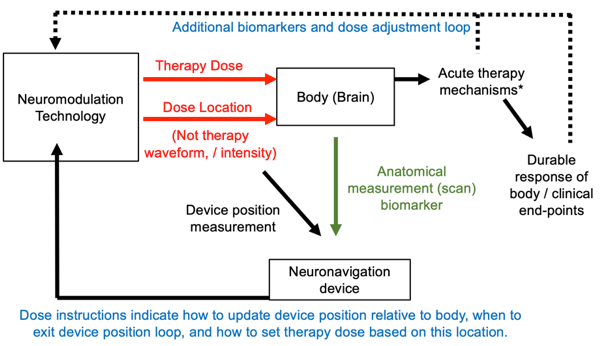
Figure: Dose tuning loop using neuronavigation as a predictive biomarker. An optional second tuning loop using a further biomarker is also shown (dashed line) of second type.
Some predictive biomarkers adjust the timing of stimulation with dose instructions as trigger function, this is gating stimulation. An example of a gate biomarker, taVNS, is gated based on breathing (PMID: 22568773; PMID: 30941526), so that stimulation is provided during specific phases of breathing. There is no expectation (as implemented) taVNS will directly change breathing. Another example is heart rate (EKG) gated TMS, where TMS is not expected to change the EKG. A further example is stimulation to aid memory where stimulation is gated to times where the brain is recorded (and decoded) to suggest a memory lapse (PMID: 28434860). In each these cases the predictive marker is a functional measure that is fluctuating with time, and it informs when stimulation should be applied. In these cases, the dose optimization loop is cycled quickly.
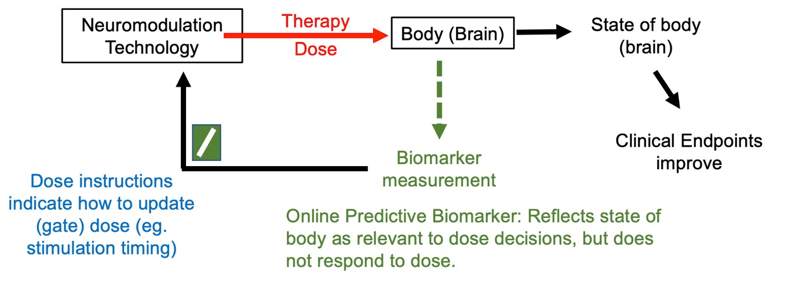
Figure: Basic dose tuning loop using online gate predictive biomarker. The predictive marker, by definition, does not change directly in response to stimulation but measures a functional or anatomical feature of the body that fluctuates during therapy and impacts dose selection.
Predictive biomarkers are defined as biomarkers that do not response to the therapy dose. However, there may be biomarkers who response is measured by applying a stimulation test dose to the body that is different than the therapy dose –these I call evoked predictive biomarkers (see figure below). This approach is common in the installation / planning phases of many forms of neuromodulation. The same device may be used to apply the test dose and the therapy dose. The therapy dose must be informed-by but be different from the test dose – a change in amplitude, location, and/or waveform.
Consider TMS for treating depression. Conventional dose instructions start with adjusting TMS dose intensity over the brain motor region until an involuntary finger twitch is produced. Evidently the finger twitch does not indicate if a person is depressed (it is not a response biomarker of the first type, a clinical surrogate) nor does the finger twitching improve mood (it is not a response biomarker of the second type, a mechanism). The finger twitch is instead a way to titrate the dose to ensure the appropriate intensity is being delivered to the brain. Once therapy begins, the therapy dose is not applied to the motor region, but instead to a frontal brain region (where finger twitch responses are not produced). Moreover, the therapy dose has a different waveform from the test dose, with therapy dose using quickly repeated (repetitive) pules. There are thus no finger twitches during the therapy dose. The finger twitch from TMS is thus an evoked response to a stimulation with a test dose, that is then used to determine a related but distinct therapy dose for each individual. The tuning loop is thus applied to the test dose and then therapy dose as shown below.
Dose instructions using predictive evoked biomarkers must distinguish the test dose from the therapy dose. For some techniques the differences between test dose and therapy dose are substantial, though the former guides the latter. For other techniques the difference may be subtle, such as an incremental change in amplitude. Dose instructions must always explain how to adjust the test dose to the predicted evoked biomarker and then when/how to set the therapy dose (figure below). To be useful, a predictive evoked biomarker must indirectly personalize the therapy dose through the test dose.
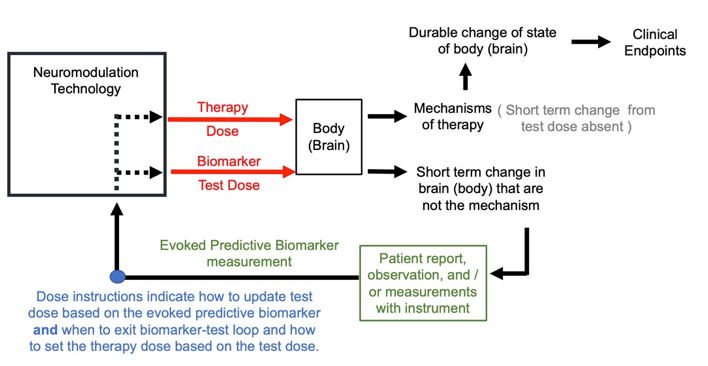
Figure: Dose tuning loop using evoked predictive biomarker.
5. Mechanism theories in neuromodulation design
I described how in SCS for pain the biomarker used for dose tuning is tingling (paresthesia) felt over the painful region; this was the presumed mechanism, so as a responsive biomarker type 2. Specifically, the non-painful tingling sensation masks the pain based on the gate theory mechanisms. Maybe. Maybe not. A new generation of SCS devices control pain without ongoing paresthesia. Nevertheless, this “sub-paresthesia” SCS use paresthesia in the process of individual dose optimization: first a high stimulation amplitude that produces tingling is used to adjust dose location, and then once therapy initiates, the stimulation amplitude is turned down below tingling levels. The dose instructions thus use tingling sensation in a test dose optimization loop, the result of which informs a non-paresthesia therapy dose – this is an evoked predictive biomarker. That pain relief occurs without tingling suggest the gate theory may not be the mechanism of SCS therapy even when tingling is present. Should this be the case, does it muddle classification of tingling in SCS as a responsive biomarker type 2 versus an evoked predictive biomarker?
It does not. The biomarker classification developed here accounts for inevitable changing ideas on mechanisms in exactly so far as these ideas change treatment technique (the loop dose instructions). So long as tingling is assumed a process essential in a dose effective for clinical therapy, dose instructions are based on responsive biomarker type 2. If tingling is not assumed a mechanism of therapy per se, but dose instructions none-the-less require therapy dose based on tingling, it remains essential in technique and so a responsive biomarker type 2. When tingling is considered in test dose adjustment, but the therapy dose is distinct from the test dose and without tingling being essential (or rather intentionally avoided), then tingling functions as a predictive evoked biomarker.
In the case of ECT for depression, where the time of pulsing is increased (number of pulses) until an adequate seizure is produced, the question of if seizures are an essential mechanism of action (the seizure initiates a therapy process) or a side-effect is an important question (PMID: 37488281). But so long as ECT dose instructions require the generation of a seizure, then seizures are a responsive biomarker type 2 (i.e., so long as seizures are inevitable following an effective dose, then an effective dose necessarily produces a seizure).
A concept for the role of mechanisms in neuromodulation development can be summarized:
The clinical benefits of a neuromodulation therapy are based on a device and associated dose instructions, not on the prevailing theory (belief) on mechanisms. Mechanism theory matters by informing the design of new devices and dose instructions (dose optimization loops). Thus, belief about mechanisms inspires neuromodulation therapy design, but the technique itself (its reproducibility) is based on dose instructions that do not require referencing a given mechanism. The use of a biomarker in dose instructions does not establish, or even imply, the biomarker is a mechanism – rather the dose instructions were inspired by an associated theory of mechanism.
This view of mechanism theories as inspiration for new device and dose instructions, but otherwise not factors in device efficacy, clarifies some prior ambiguity about the role of biomarkers as mechanisms as well as about the role of mechanism research in clinical trials.
At first blush one would think: if it successful to titrate neuromodulation therapy dose based on a biomarker, this implies the biomarker is (or at a minimum tracks) the mechanism of therapy. As explained here, there are biomarker types which are assumed unrelated and even orthogonal (unchanged by stimulation) to therapy mechanisms. Even when a biomarker is assumed a mechanism of action (used as a type 2 responsive biomarker) and treatment is successful, the biomarker may not be essential and so not a mechanism. To show clinical success, a useful biomarker need only accelerate the process of dose optimization.
Clinical trials are costly in financial, human, and time resources. Moreover, results from clinical trials may be not definitive or not reproducible (at larger scales). Motivated by accelerating treatment creation, the National Institutes of Health (NIH) developed funding strategies prioritizing clinical trials with “target engagement” biomarkers. Sometime defined as a “factor that is hypothesized to mediate the intervention’s effect” or
“…demonstrate that the intervention exerts some measurable effect on a hypothesized “target” or mechanism of action; the intervention is used as a manipulation with the immediate goal of determining whether the intervention affects a target rather than attempting to demonstrate a clinical treatment effect. “Target engagement” refers to verification that the intervention has had the predicted effect on the target. Targets may be molecular, cellular, circuit, behavioral, or interpersonal, commensurate with the intervention. Once target engagement is demonstrated, measures of target engagement are then related to clinical outcomes to test the hypothesis that the target is relevant to the clinical problem under study.”
NIH dominates public support of medical research, and sets a culture across funding and review institutes, so that mechanism theories are now broadly hitched to clinical trials. It is certainly rational to link development of treatments to understanding their underlying mechanisms. But clinical treatment may be effective with a wrong mechanism theory. And both mechanism theories and treatment techniques are always evolving. To define “target engagement” as a change in the “target” may miss the distinctive relevance of biomarker types (in dose optimization). The concept developed here, whereby mechanism theories serving to inspire biomarker driven neuromodulation, provides a functional and practical link between theory of mechanism and treatment design.
Far from considering mechanisms incidental, my approach puts mechanisms central to innovation. “Modern” neuromodulation started with the development of device and dose instructions from evidence-based (though imperfect) theories of mechanism. As opposed to approaches inspired by meta-physical notions of physiology / stimulation mechanisms. We can never have a perfect (true) mechanism of neuromodulation as that implies a definitive understanding of brain function/disease, the underlying biology and overlying behavior/psychology, and exact biophysics of stimulation. Our understanding of such things is incomplete, certainly riddled with inaccuracies, but there are still frameworks of understanding. Frameworks that are enhanced through new science. The instructions for dose optimization are based on a theoretical framework, e.g., gate theory for pain or the role of the role of frontal cortex activity in mood. Without some theoretical framework we have no rational basis for suggesting how to use biomarkers. And supposedly the better framework we rely on, the better dose instructions based on biomarkers we can developed. Since no framework is perfect, some intuition (and luck) is involved.
In designing how to use biomarkers in neuromodulation, theoretical mechanism frameworks have three components (figure below). First, how dose (energy applied to the body) produces changes in physiology and then behavior and clinical endpoints. This has been referred to as “Input/Output” (I/O) modeling, where the input is the dose and the output the body response of interest. One might think knowledge of just this first component would be enough, except that the effects of any given dose (the I/O) will depend on the state of the body, which is individual and changing. The second component is measuring and analyzing the state of the body as relevant to guide dose selection. In principle this can be any objective or subjective measure. There are inherent challenges with all measurement techniques including the accuracy of the measurement apparatus and relating the measurement to a ‘deeper’ body state. This can be called “decoding” the measurement. The third component is how to update the dose based on the biomarker, which must involve considering both the I/O and decoding theories. Neither the I/O or decoding components are complete or necessarily correct, which is why there is “art” in the third component. This can be called “control systems”. The control system might consider, for example, if there is a delay between an updated dose and change in measured biomarker.
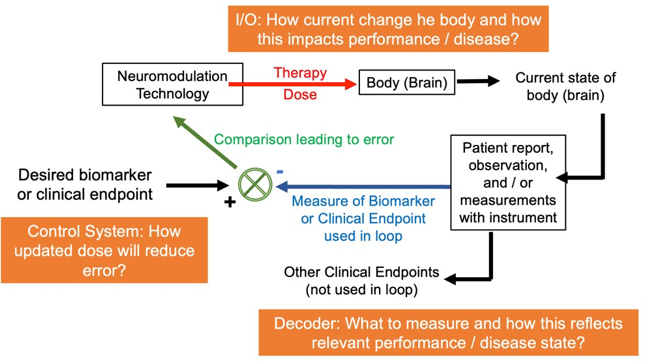
Figure: The background knowledge that informs dose instructions has three components: I/O, measurement/decoding, and a control system. Dose instructions are practical rules that collapses across all three components, but each component can be independently researched and optimized.
The control system in fact compensates for unknowns. Consider the analogy of a thermostat. The thermostat measures temperature in one corner of the room. It then controls the activation of a heater or air conditioner somewhere in the room. How much the room heats/cools depend on many factors (unknown to the thermostat) such as the heater/cooler power, outdoor temperature, or if a door is open. Nonetheless, though loops of measuring temperature and adjusting heating/cooling, the temperature in the room may be well regulated.
Like a thermostat, the simplest control system compares the measured body/brain state against a desired (goal) body/brain state. This comparison, which can be a simple as subtracting one from the other, produces an error between the measure and desired state. Zero error suggests the measured state is the desired state, while a large error suggest the measured state is far from the desired state. The dose is then updated based on this error; for responsive biomarkers with the aim of reducing the error - bringing the measured state closer to the desired state. Depending on the biomarker type and associated loop implementation, the error can be of a clinical surrogate, an assumed mechanism or energy dose, a feature of a body signal, or something else. The design of a control system and its associated loop structure, depends entirely on (physically embodies) the theory of the treatment.
Dose instructions collapse across all three mechanism framework components (I/O, decoder, control system) in the sense that the user is just provided with rules to follow – the mechanisms in each framework component are only implicitly in dose instructions. But it remains useful to separate each mechanism framework component, as each can be developed (researched) on its own. Or put differently, when treatments do not succeed as hoped, we can search for errors in our understanding of the I/O, or the decoder, or control system. Correcting such errors in the background theory can in turn lead to new design of better dose instructions.
Mechanism theoretical frameworks are developed around the use of biomarkers to individualize neuromodulation. There is no single “leading” framework. Nor is any one framework strictly defined. But the framework is the background knowledge from which novel devices and associated dose instructions are conjectured. And so, these devices and dose instructions are embodiments of the theory of mechanism, including what/how biomarkers are used. The clinical success of a treatment (clinical trials) provides indirect confirmation or refutation of the theoretical framework, leading to updates in the framework. Updates in background theory in turn suggests new device and dose instruction designs. A big framework-development loop around subordinate dose-instruction loops. This big loop is precisely how knowledge is built with the goal of ongoing neuromodulation therapy improvement - which is explained in the remainder of this section (and shown with examples in the next section).
While I suggested three component of background theory in the context of dose instructions (figure above), and describe device technology and dose instruction as the embodiment of mechanism theories, it is important to emphasize there is a leap from mechanism theory to a neuromodulation system design. There may be multiple (and even competing) neuromodulation technologies or dose instructions derived from the same mechanism theories. And distinct mechanism theories leading to the same device implementation. Hence mechanisms theories are only implicit on neuromodulation systems.
A note of causality versus correlation, that has led to mistakes. One can establish a causal relationship between a stimulation dose and a biomarker (such as presence paresthesia with sufficient dose amplitude). And one can separately establish a causal relationship between stimulation dose and the clinical endpoint (such as pain reduction with sufficient dose amplitude). This does not however establish a causal relationship between the biomarker and the clinical endpoint (such as paresthesia and pain reduction). Since conditions may exist where the biomarkers it not at a target level, yet clinical benefits results (such a parathion-free pain reduction).
Even a type 2 responsive biomarker is only assumed a mechanism of action. And it is because new evidence can change our understanding of a biomarker (e.g., a biomarker is predictive rather than responsive) that even successful clinical treatment with biomarker loops does not establish a causal role for the biomarker. In many cases there is no no risk of confusing an effective dose instruction using a biomarker with establishing the biomarker as mechanism (e.g., finger twitches during TMS adjustment are not themselves therapeutic), there are many situations where the significance of this distinction not recognized – including in pressure to prove “target engagement”.
Given the therapeutic usefulness of a biomarker does not establish it as a mechanism, how do background mechanistic theories underpin inventions using biomarkers? The background mechanistic framework is conjectural, a best-guess that inspires practical dose instruction. The centrality of biomarkers is implicit to them underpinning dose instructions. Should the dose instruction not prove successful in treatment, such a clinical result may refute the mechanistic framework or its translation into dose instructions. This encourages a revision of the mechanistic framework and then updated dose instructions. Conversely, if a mechanistic framework suggests a dose instruction, that is in turn proven successful in treatment, the mechanistic framework is not refuted - but it is not proven true. Scientists will recognize this standard paradigm of theory (hypothesis) testing, where the testing of dose instruction is the experiment to refute a theory.
So, the device and dose instructions are the physical embodiment of the background mechanistic theory, and a clinical trial using the device and dose instructions therefore tests the falsity of theory (alongside the more evident purpose of establishing clinical efficacy). Through ongoing successful and failed clinical trials, this process is repeated, forming a mechanism-driven design loop:
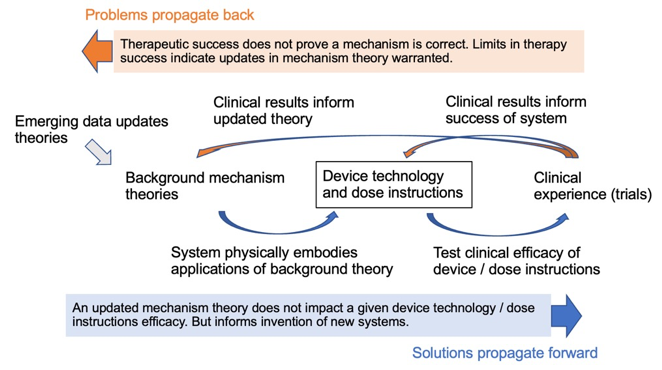
Figure: Background mechanisms theories inspire the design of devices and dose instruction, which are in turn tested in clinical trials. Results from clinical trials reflect the performance of the device and dose instructions and so on the underlying mechanism theory. Mechanism theories will be largely formed through emerging data unrelated to the clinical trials. There is a “forward prefigurated” therapy development/validation pathway and a “back propagated” pathway for corrections/updates.
There is thus a therapy development/validation pathway: from a background mechanistic theory, to a device/dose instructions embodying that theory, and then to a clinical trial testing the device/dose instructions. In the limited context of this pathway, any success of the clinical trial reflects on the validity of the mechanistic theory. But the mapping from the “truth” of the theory, to “uniqueness” of the device/dose-instructions, to the “design and interpretation” of the clinical is by no means definitive. The same theory may lead to the design of distinct devices, or distinct theories may lead to an identical device. And clinical trials may success or fail for a host of reasons. Background mechanism theories will be updated based on convergent factors, irrespective of new clinical results with devices they inspire. Nevertheless, clinical trials must be of specific device/dose instruction. And devices/dose instructions are inspired by a given mechanistic theories (theories which at some level look forward to the clinical application). This is what I mean by solutions propagate forward. When a clinical trial succeeds or fails that result reflects on the devices/dose-instruction tested, and through the technology to the background mechanisms theory. And failures inspire changes. This is what I mean by problems propagate back.
With the role of background mechanism theories in device design thus clarified, we can develop a criterion for impactful research of neuromodulation mechanisms. If a theory (or new method of analysis) is developed to explain the outcomes of an existing technology and dose instructions, without suggesting a change to either, then that new theory has limited immediate impact on device design and so outcomes. Conversely, an impactful theory (and this can be an impact for good or bad), suggests new technology or dose instructions. As the saying goes: “A difference, to be a difference, must make a difference.” A new theory does not change the efficacy of any existing technology and dose instructions, and so for this reason it is possible to “back out” (reverse direction of the development/validation pathway) from any neuromodulation system/clinical outcomes new theories – but with limited impact. New mechanisms theories that are not immediately impactful may become so, if they are then recognized to suggest new neuromodulation system designs.
In this context, research on the etiology of disease or (preclinical) disease models impact therapy development when discoveries inform technology/dose instruction development (e.g., theory of mechanism whereby stimulation reverses a pathological process). Otherwise, research on disease is incorporated into general background theory, pending recognition how it might inspire technology/dose instruction design. Similarly, in this context we can emphasize basic science on stimulation mechanisms (e.g., which neuronal elements are polarized) that directly inspires new technology/dose instruction development (which can they be tested in clinical trials) versus research that stacks onto general background knowledge. The saying goes “A difference, to be a difference, must make a difference”.
Consider the example of ‘virtual lesions’, a background mechanism theory that suggests certain neuromodulation doses will disrupt function in a brain region, with functional outcomes analogous to lesioning that brain region. The disruption lasts only as long as stimulation, making the ‘lesion’ only ‘virtual’. This virtual lesion mechanisms framework, applied to diverse neuromodulation technologies, inspired a range of treatments and cognitive neuroscience experimental interventions. The dose location is applied a brain region to be virtually lesioned. The success of such approaches in trials, as measured by behavioral outcomes, is consistent with the virtual lesion theory but does not prove it – rather the virtual lesion theory avoided falsification by these human trial results. In some cases, the virtual lesion theory was discovered through additional experiments, such as those measuring brain activity, to be inadequate (PMID: 19166996; PMID: 17945512; PMID: 11948756; PMID: 14668299). This constitutes new emerging background data that can be used to update the mechanistic theory, but this does not mandate new devices/dose instructions, and more than such new background knowledge invalidates the success of human trials. The revised non-virtual-lesion theories matter for therapy optimization when they suggest dose optimization divergent from virtual lesion theories.
The mechanistic framework can be as generic as a rule-of-thumb, or it may specifically reference any scale of processes (from molecular to psychological). For example, the framework may posit that applying energy into the body “boosts function” which in turn can, in principle, treat any disease marked by “hypo-function”. Or that energy enhances “plasticity” and so can boost, in principle, any type of neurorehabilitation or cognitive/behavioral training. The generic framework may be connected to cellular theories of neuronal function or plasticity. Does this mean any mechanistic theory (including the most hair brained) suffices to inspire the design of a neuromodulation devices and dose instructions? It does. But inevitably, there will be failures testing the devices/dose-instructions on some patients. To learn from this experience, one propagates the failure back to the device/dose-instructions and then to the mechanism theory. If the mechanism theory is so ill-defined or ‘squirmy’ that is withstands any falsification, meaning either consider the clinical results irrelevant to the theories validity or invent ad hoc rules to protect the theory, then the theory is not updated. With a stagnant theory, there is no inspiration for updating the device/dose-instructions. So, no progress. Mechanisms theories should be falsifiable. Mechanism theories need to take ‘risks’ in the sense they don't allow everything. Theories that can dodge falsification (but accounting tor any possible result) were referred to by the philosopher Karl Popper as ‘meta-physics’.
The concept of device design loops also provide context on the role of device technology (e.g., electronics, accessories). Dose instructions select from a possible dose set available through the device, and so evidently a dose a device cannot produce cannot be selected from. For example, a device designed for implanted electrode locations (implanted) cannot provide stimulation with electrodes on the skin (non-invasive), and vice versa. No device allows all combinations of amplitude and waveforms/timing. There are many reasons of limitations set by manufacturers based on usability, safety, cost, complexity, lack of efficacy, or legal/regulatory concerns – these factors are intertwined. New background knowledge can impact any of these, which in turn motivates devices with expanded dose sets (e.g., a new frequency) or device features not specific to dose (e.g., new battery). Once built, the new devices can be tested. Those tests may show the value of the new doses (e.g., better treatment with a new frequency) and of non-dose related features (e.g., longer battery life). Biomarkers play a central role in dose-related design as dose instructions depend on biomarkers.
The central role of biomarkers reflects them governing neuromodulation dose instructions (i.e., dose selected for to an individual from the device technology). To the extent personal titration is ubiquitous in neuromodulation, so does neuromodulation success hinge on biomarkers. Conversely, cases of limited success of a neuromodulation approach can be attributed to a failure to implement these loops well, by which I mean both the inner biomarker-based dose instruction loops and the outer mechanism-based intervention design loop.
I recognize my method of describing neuromodulation design / optimization is novel and so maybe foreign or surprising to those working in the field. But my explanation is entirely descriptive of existing practices - albeit with a new categorization system of biomarker loops – not inventive of new ones. Much work on neuromodulation optimization may seem to fall outside of the biomarker loops described here – and this work can be considered part of the creation of background information. For example, post-hoc analysis of neuromodulation outcomes considering if a biomarker stratifies response may be used in future trial where the biomarker is in fact used to adjust dose. Any studies on pre-clinical models, if translatable, may inform dose tuning loop – for example by identity a candidate biomarker. A large number of studies considering individual responses (variability) to neuromodulation entirely rely on outcome measures distinct from behavioural endpoints. For example, many experimental NIBS studies effects on TMS-MEP, as end in itself. Even when such experimental studies tune dose, they are doing so without concurrent tracking on a behavioral endpoint – and therefore there is gap between their results and evidence for application-based dose tuning. None the less, such experimental studies are foundational to neuromodulation therapy development. As described elsewhere in this document, studies on closed-loop or computer modeling technology can fall withing the biomarker dose tuning loops described, or in other cases may be part of general knowledge creation (e.g., making models faster or more accurate). Any research work on technology such as electrodes or coil shape, stimulator performance, sensors etc. is essential but distinct from therapy implementation. All these activities that enhance background knowledge are shown in the figure below.
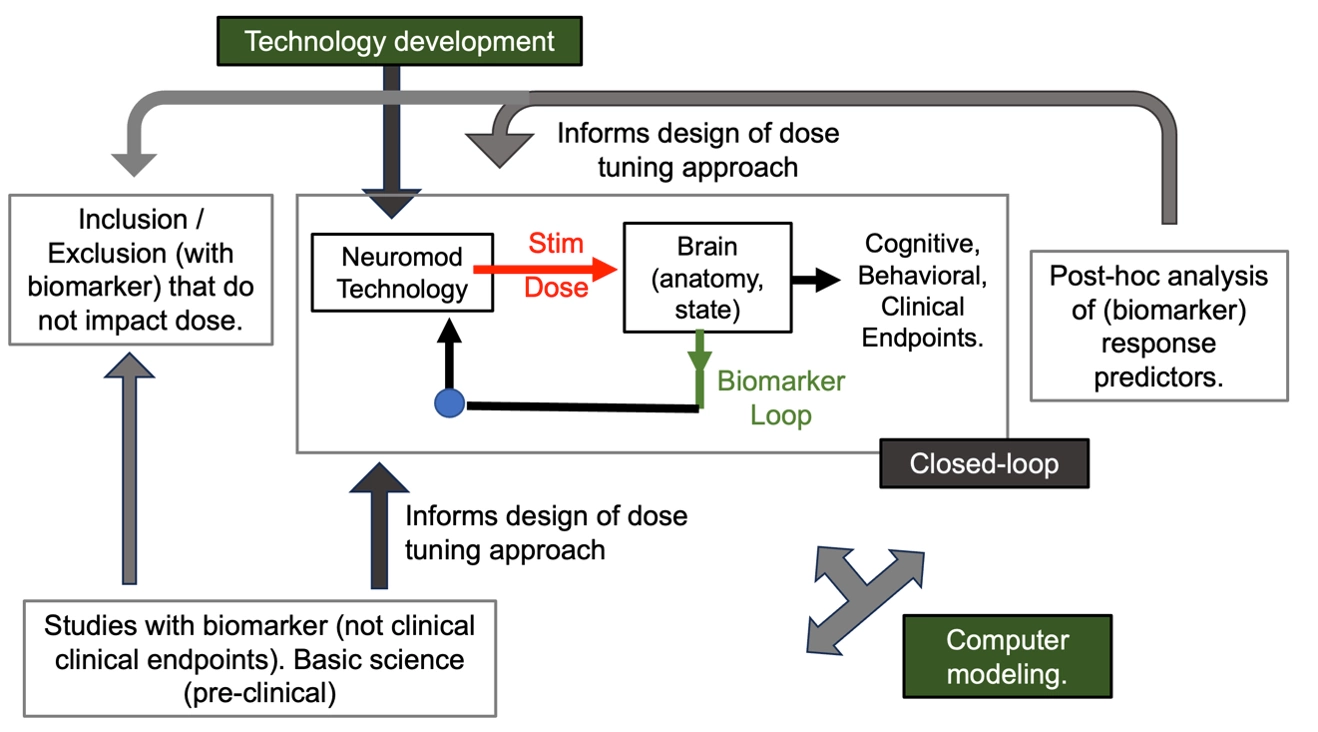
Figure: Research activities that develop background knowledge. Such activities are distinct from therapy dose-optimization loops, but can inform their design
6. Reproducibility
The history of neuromodulation is almost as long as (and its developments often juxtapose against) pharmacotherapy. As with any therapies, there are neuromodulation devices whose popularity has crested and fallen, though we see their (indirect) decedents in other modern approaches (PMID: 23954780). There are varied medical and non-medical reasons for a therapy’s success, but reproducibility is overarching. One cannot establish (or clearly disprove) the efficacy on any treatment, is the treatment itself is inconsistent in how it’s built or used. In neuromodulation, these are, respectively, device technology and dose instructions.
I expect historical devices like the Electreat and approaches like Electrosleep therapy worked for some patients some of the time, and in these limited cases provided real benefits. But to reproduce that success among other patients, with different care-givers, across decades, requires consistency in key aspects of device technology and dose instructions. When either is under-specified, variations are inevitable, and outcomes become muddled.
In device technology, we distinguish between those features that relate to dose (amplitude, location, waveform/timing) and all other aspects. Dose instruction guide selecting a dose from the set possible with a given device technology. If device technology relating to dose changes, so does the set of possible doses. Not all changes will significantly affect outcomes, but at a minimum new device technology (doses) must be acknowledged and considered in any attempt to consolidate experiences.
For a therapy to be reproducible, dose instructions must be explicit. This can take the form of a manual provided with the device, regulations or standards, and/or formalized training and certification procedure. What details of a therapy protocol should be included in dose instructions? All details that impact what dose is ultimately provided.
One can argue: surely factors outside of dose, and so dose instructions, impact treatment effectiveness and so must be considered? This is correct. For example, a fault in device design or procedures that impact safety (unrelated to dose) is evidently important. Or the use of adjunctive therapies (e.g., pharmacotherapy, rehabilitation, cognitive therapy) with neuromodulation is important for overall outcomes. The emphasis on device dose and dose instructions for reproducibility is not in opposition to controlling other factors in treatment. But underspecified device dose and dose instructions a priori undermines all efforts to validate and scale neuromodulation.
What about the “art” of medicine, and the expectation that skilled caregivers adjust a therapy to a patient’s unique and evolving situation? We care about selecting our health care providers for our expectation they do provide such specialized treatment. In neuromodulattion treatment health care providers are selecting dose (location, intensity, waveform/timing) from the options provided by a given device – and are doing so according to dose instructions. Here “art” indicates skill in optimizing dose in a way that is no generic (following a strict rule-book) perhaps because dose instructions are underspecified for the given patient’s needs, or the caregiver improvises beyond documented dose instruction. If that special technique is not rigorously document and transmitted to others, then in remains the “art” of the specific care-giver (it cannot be reproduced by others). Only if the caregiver documents their new dose instructions, then the new neuromodulation treatment that can now be reproduced. In fact, this process of invention through clinical experience and then publication through clear dose instructions is precisely how clinical scientist play a central role in neuromodulation invention.
By what process do clinician scientists arrive at neuromodulation inventions? First, they encounter a problem (a limitation) in existing care, which being at the front-line of medicine they have unique perspective of. Second, they conjecture a new device or dose instructions. Given infinite variations of dose, how do they suggest a specific new approach to dose? By referring to their background knowledge which includes theories of mechanism – these may be detailed or “rules of thumb”, but must explain how to change at least one aspect of dose. If a biomarker for dose tuning is proposed, then the biomarker type governs the structure of the dose optimization loop. Third, a new device or dose instructions is developed and tested, whose success is only indirectly dependent on the accuracy of background mechanism theory.
Discussing (proposing) a mechanistic theory does not excuse providing complete details on device design and dose instructions. Confusing conjectures of mechanism with dose control is indeed a common error of pre-modern neuromodulation. For example, the Electreat device marketed “Two things cannot occupy the same space at the same time. Shooting electricity in forces the pain out.” But other than placing the device over the painful region, no dose details were provided.
When a biomarker is used to guide dose selection, it is implicitly understood that biomarker relates to the theory of treatment mechanism. But dose instructions should explicitly reference the biomarker, not the mechanism theory. This is true even for a type 2 responsive biomarker which is assumed to measure a mechanism. Any mechanisms theory may be revised (indeed changes are expected) without impacting the reproducibly of the original biomarker-based dose instructions.
The dose instructions for use should be explicit, such as a manual provided with the device. Inexplicit dose instructions (art) inevitably arise, through mentoring of practitioners or anecdotal experience with patients. The risk of inexplicit dose instructions is limited reproducibility. Patient 1 with symptoms X1 may have responded well to treatment from device Q1, following personalized adjustment by dose instructions D1, applied by Doctor A. Later Patient 2 with symptoms X2 is treated by doctor B with device Q2, with personalized adjustment by dose instructions D2, but does not respond. Differences between symptoms X1 vs X2, device Q1 vs Q2, or dose instructions D1 vs D2 explain differences in outcomes. But if dose instructions D1 and D2 are not fully documented (or even explicitly controlled), we are at a loss at the start of this analysis. Similar problems arise is differences between device Q1 and Q2 are not known. Without reproducibility in device dose and dose instructions, we are doomed to faltering progress in treatment discovery.
Reproducibility underpins the development of any therapy. For each type of therapy, there are specific factors most important for reproducibility. If dose tuning to individuals is essential for a given neuromodulation intervention effectiveness, then:
1) Neuromodulation effectiveness is governed by the details of device build as impacting dose and the dose instructions whereby biomarkers are used in dose optimization loops (notwithstanding how non-dose factors also contribute).
2) Theories of mechanisms inspire, but are not inherent to, device or dose instruction design. These theories including how stimulation changes the body, the theoretical relevance of biomarkers, and why specific control schemes are effective. Discussing theories does not excuse not providing sufficient detail for device technology / dose instruction reproduction.
3) Lack of appropriate detail (or even control) in device build as impacts dose or in the dose instructions limits reproducibility, and so the replication and expansion (across centers and long period of time) of any clinical results. Irreproducible dosing undermines the value of any clinical trial and acceptance of any neuromodulation intervention - if not setting up its eventual abandonment all together.
7. Examples, Loop implementations
In developing a biomarker classification scheme, I aimed for a clear description that was complete and internally consistent. My approach was descriptive of existing approaches, and not prescriptive of new approaches. Specific dose instructions loops should seem familiar (even described too simply) to its practitioners – but I hope it is insightful how similar elements are applied across different dose instructions loops, aligned to biomarker types. And I hoped to enhance existing schemes such as NIH-FDA BEST that were not developed to distinguish neuromodulation approaches. Some additional comments on completeness and consistency are useful.
The NIH-FDA BEST guidelines and associated commentaries (PMID: 29405771) have some differences with the classification developed here. Exclusion / inclusion criteria and ‘diagnostic biomarkers’; are not biomarkers as defined here, unless they are explicitly used in dose instructions; while such criteria may be predictive of response to therapy, that may also be based on non-therapeutic factors (e.g., subjects excluded from trials for cost reasons). In NIH-FDA BEST context (PMID: 29405771) “biomarkers should be distinct from direct measures of how a person feels, functions, or survives—a category of measure known as a clinical outcome assessment (COA)”. However, the approach here is based on neuromodulation indicating all objective/subjective measures used to tune dose are biomarkers, and clinical endpoints as separate. NIH-FDA BEST has classifications like a “monitoring biomarker” (something measured repeatedly) which is acknowledged to be broad and overlap with other biomarker categories. Thus the deviation here from some prior practices reflects my goal to develop and cohesive classification that directly maps into neuromodulating practice, specifically dose instruction loops.
Let’s say one is recording beta oscillations from a part of the brain as part of a neuromodulation treatment protocol, and using the recorded brain location, frequency, and/or magnitude of the oscillations for dose optimization. If the oscillations are a marker of the disease (e.g., their magnitude correlated with symptom severity) it is a clinical surrogate, so a responsive biomarker of the first kind. One expects that as the symptom’s changes so does the biomarker, and in a durable way. If changing oscillation activity is the therapy mechanisms (but not a clinical surrogate) than they are a responsive biomarker of the second kind. In this case, the oscillations may change transiently during effective stimulation, but do not show a durable change correlated with slow symptom improvement. If stimulation is applied at individual recorded oscillation frequency, but oscillation does not change in response to stimulation (as far as dose instructions are considered), then oscillations are a playback predictive biomarker. If neuromodulation dose is tuned considered how oscillations change to a test dose but the final therapy dose does not (as far as dose instructions are considered) change oscillations, oscillations are an evoked predive biomarker.
So, we see that the same measurement (in the example above oscillations) can function as different types of biomarkers. In the scheme developed here this is not a source of ambiguity but rather follows directly from a classification method based on presumed biomarker function and associated loop implementation. Each approach leads to distinct dose instructions (loop implementations) and so each approach can result in distinct dose-selections. The outcomes, and so reproducibility, of neuromodulation depends on 1) device build and 2) dose instructions. So, what one assumes about the significance of a biomarker marker exactly so far is it determines the dose instructions – how we implement the optimization loop. And so, for the same reason, the development of a new neuromodulation therapy is centered on device build and dose instructions. Mechanism discovery and verification are conspicuously absent in this specification of neuromodulation therapy, except as they inspire suggest the design of dose instructions. Theories about mechanisms can ‘accidently’ inspire therapies: they may be false and the resulting therapy still technique to be clinically effective.
Two hypothetical studies examine the effectives of neuromodulation for a brain disorder while simultaneously measuring change in a brain signal. Both clinical symptoms and the brain signal were measured at the start and end of treatment. The brain signal was not used to adjust dose. Both studies reported improvement in clinical symptoms, with one study showing improvement was associated with a brain signal change, and other showing brain signal changes were not correlated with clinical improvement. In neither case was the brain signal a responsive or predicate biomarker, as it was not used to adjust neuromodulation dose. One trial confirms the brain signal might be used a type 1 responsive biomarker (clinical surrogate), the other refutes this. These trial outcomes do not evidently inform if the brain signal can or cannot be used as any other biomarker type – though reanalyzing the trial results from a different perspective, the signal may. The point is that in developing background knowledge to design closed-loop neuromodulation, biomarker types need to be distinguished.
A further example of how a biomarkers significance depends on theory of mechanism. A Responsive Neurostimulation System (RNS) is an implanted device intended to treat seizures; it does so by measuring ongoing brain activity and when detecting an atypical providing responsive stimulation. Success is measured in part by reduction in the atypical brain signals, which are considered direct surrogates for clinic seizures – making the biomarker a responsive type 1. But there is nuance. There may be two types of atypical brain activity. The first is a pre-seizure buildup, but not a seizure per se. The second being the seizure. To the extent the RNS is measuring and inhibiting the pre-seizure buildup (rather than stopping seizures as they start) than it is using a responsive biomarker of the second kind: The mechanism theory is the reduction in pre-seizure activity. As a further alternative, what if the pre-seizure activity is modulated by the RNS system when the dose is well optimized, but RNS suppresses seizure through a mechanism not related to pre-seizure activity? For example, the brain activity has a random nature that when analyzed suggests a RNS with a random pattern that is coincidentally suitable for seizure control. If one adjusts RNS to provide pre-seizure inspired random pattern irrespective of if pre-seizure activity changes, then that activity serves as a predictive biomarker. Recognizing distinct biomarker types, results in distinct dose instructions. It is possible that distinct dose instruction produces, in some cases, the same dose (and so outcomes), but this is no justification to muddle them. Progress toward better dose optimization depends on rigor (not ambivalence) in technique.
Suppose the RNS company provides three software versions (dose instruction) of their product based on: option A assuming activity tracks seizures (responsive biomarker type 1); option B assuming activity is a pre-seizure build up (responsive biomarker type 2), or option C assuming activity can be used generate pseudo-random dose timings but it not otherwise important (predictive playback biomarker). The RNS hardware is implanted in a patient and each software version is tried in turn. Further suppose it is discovered that in all these options the device output (dose) is the same - which also means expected therapeutic outcomes are the same. Between option A and option B, this is expected. The dose instructions can appear the same - only the underlying type (type 1 surrogate versus type 2 mechanism) varies. For option C this is possible provided certain features of the activity and dose instructions. If the dose in this subject was the same across all three options, and so the therapeutic results the same, does that means the varied dose instructions is a difference without practical significance? Such an attitude is faulted. It disregards the modern approach of developing mechanism-based neuromodulation techniques - since it adopts ambivalence about the mechanism. It undermines reproducibility. Option C may produce a distinct dose (compared to A/B) in another patient, or the same patient at a later time. What is learned from the success of each option, as well as general growth in backroad knowledge, can inform design of improved dose instructions. For example, based on option B, it may discovered that an optimal dose should not suppress pre-seizure activity so much as ensure is does not transition to a seizure. Mechanism theories, albeit imperfect and changing, underpin development of better technology. That’s true in designing better airplanes, running shoes, and toasters - so for neuromodulation as well.
As already suggested, biomarker types are not exclusive for each therapy; or one can run loops in alternating or parallel fashion. The thing to keep straight however it that each loop is based on a biomarker and dose instructions appropriate to that biomarker type. In principle, a given loop may combine multiple biomarkers if they are of the same type.
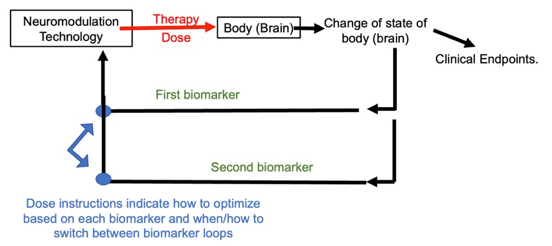
Figure: Two biomarkers, two loops
Loops can be nested so long as dose instructions indicate how to exit and enter another loop. Moreover, the result of implementing one loop can be used to update the dose instructions of another loop. This is perhaps more common with clinical endpoints. Depending on how clinical endpoints change with given dose instructions, one could update all underling loops. We can consider two examples.
In one example, the dose instruction for an evoked predictive biomarker loop may be updated based on clinical experience (Figure below). A patient receiving TMS for depression has for each session a motor twitch from a test dose is used as a predictive evoked biomarker and a therapy dose set based on the test dose. Dose instructions indicate how this process is implemented. If it discovered a patient is not responding clinically, one could than develop new dose instructions of how to set treatment dose based on the test dose.
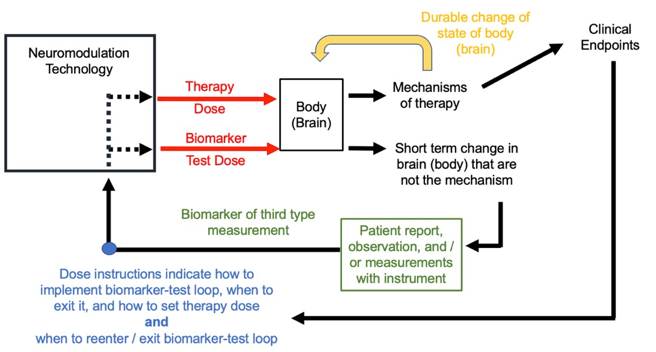
Figure: Two loop implementation with interaction between loops.
In another example of clinical endpoints in an outer loop that are used to guide of an inner biomarker loop dose instruction, we consider the use of ECAPs to guide SCS. ECAPs are presumed responsive biomarkers of the second type and the goal of dose optimization is to provide a dose producing an ECAP response of a sufficient size: not too small or no clinical benefit is expected and not too large or side-effects are expected (a therapeutic window). However, what constitutes a small or large ECAP can only be known by asking each patient when they feel paresthesia (the lower efficacy threshold) and when they feel discomfort (the upper threshold). One therefore needs to access patient feedback in the outer loop (which includes a clinical endpoint of discomfort), and then provide these values to an inner loop based on ECAPs that runs the ongoing therapy.
Computational models of neuromodulation can be used in three stages: 1) in analysis of collected data for the purpose of enhancing background theories (not in the process of system design or treatment); 2) in the use of background theories to design device technology / dose instruction (before treatment), which can be called computer driven design; or 3) as part of the dose instructions (during treatment). Computational models can be used in dose instructions with any responsive or predictive biomarker type. There are anatomical or functional measurements that can and used by computational models to personalize neuromodulation. Computational models based on anatomy start with individuals’ anatomical images (e.g., MRI) and simulate different device doses to select an optimal one. For example, software that includes patient specific anatomy can recommend between different electrode locations. Computational models can incorporate any additional functional measurements (fMRI, EEG). The prediction of energy generated in the body by computational model is analogous to its measurement by type 3 responsive biomarkers. Models may be extended to make functional predictions regarding mechanisms of action (analogous to type 2 responsive biomarker) or clinical endpoints (analogous to type 3 responsive biomarker). But model predictions, whether of energy, mechanism, or clinical outcome, are not measured responses. Dose instructions are rules, logical systems, algorithms - with computational models being one calculation tool. As such, computational models integrate into, but don't change, the biomarker types and loop structures presented here.
Forecasting can serve two roles in this framework. If there is a delay between the applied dose and the relevant therapy-driving changes, one needs to model such a delay in dose instructions. Conversely, the biomarker may indicate a future (delayed) change in clinical endpoints, which again would inform how dose instructions updated dose. Except for factoring in delays, don't change, forecasting does not change biomarker types and loop structures presented here.
“Closed loop” stimulation is an approach to automate the adjustment of neuromodulation dose with a biomarker. In closed loop stimulation a measurement of the body is made, for example a sensor that measures the tremor of a hand, and a computer makes a decision on what dose should next be applied based on this measurement. The key is that a computer is updating the dose, without necessary human intervention. Any biomarker / loop system described here can be applied for closed loop. Faster loops (where dose is updated in sub-seconds) require closed-loop. Now the parameters a closed-loop system uses (its programming) are adjusted by a human operator; this can be by a parameter-adjustment loop around the closed-loop. Analogous to a person manually setting the target temperature on a closed-loop thermostat. For example, how ECAP SCS is implemented (above). At a minimum, the overall operation (clinical endpoints) of a closed-loop therapy system is initiated and overseen by a human operator. Practically, we may define a closed-loop neuromodulation system as one where for at least some portion of the therapy, the dose is adjusted automatically (by a computer) using a biomarker.
The discussion above was explicit to clinical treatments, but all implicitly applicable to performance enhancement applications. There is also the use of biomarkers in the basic science of neuromodulation, or still more broadly brain science (from on neurophysiology to cognition). When the use of a biomarker is not directed for optimizing neuromodulation dose to enhance clinical outcomes, the framework here does not evidently apply. However, such research enhances the background knowledge used to explain and invention neuromodulation treatment. This is clearly the case when a dose optimization loop is developed to regulate a biomarker that has potential relevance in treatment. Brain stimulation as a research tool has, and continues to, play a central role in neuroscience – because a system can be characterized (probed) through its controlled perturbation. Discoveries across basic science will lead to new biomarker types and dose instruction loops, not considered here. Given the motivation for science is creation of new knowledge and methods, no classification can anticipate such discoveries. The scheme developed here may nonetheless help frame new approaches.
8. Summary of how biomarkers inform neuromodulation design
Neuromodulation is personalized thorough adjustment of stimulation dose; namely amplitude (in voltage or current), location (of the electrode/coil interface), and/or waveform/timing (spanning pulse shape to schedule). Two neuromodulation approaches providing the same dose are indistinguishable in their direct physiological responses. Conversely, any neuromodulation innovation must involve a least one change in dose. However, the response to stimulation dose depends on underlying anatomy and physiological/brain state. Variability in the anatomy and physiology thus leads to different outcomes.
To address this variably, neuromodulation dose can be adjusted to individuals using biomarkers. Dose instructions indicate how to adjust dose based on biomarkers, specified as titration loops. Each device, as well as any (regulatory) use standards, restrict the selectable dose range (e.g., implant electrode locations, maximum intensity) from which dose instructions select. Even so, each device technology provides many doses options, necessitating the need for biomarkers to guide dose selection.
Rational neuromodulation requires distinguishing types of biomarkers. Biomarkers may be classified practically by how they are used in dose instructions (loops). In this context, biomarkers are defined as any objective or subjective measurement from individual used to adjust dose, with the goal of improving clinical endpoints. Biomarkers may be either responsive biomarkers or predictive biomarkers.
Responsive biomarkers change in response to therapy dose in a manner that informs dose optimization to clinical endpoints. A clinical endpoint cannot be a responsive biomarker. While the classification between a clinical endpoint and a response biomarker may vary by application, it is important to not confuse biomarkers with clinical endpoints. Only improvement in the latter marks treatment’s success.
Responsive biomarkers are type 1 Clinical Surrogates (change alongside clinical endpoints), type 2 Theory of Mechanisms (change rapidly compared to clinical endpoints), or type 3 Electrodynamic (change instantly). Responsive biomarkers are measured repeatedly, and dose adjusted iteratively; dose instructions are thus a loop of biomarker measurement and dose tuning. The structure of the dose instruction loops is similar across responsive biomarker types except in their presumed time course relative to clinical endpoints (Figure).
With a type 1 responsive biomarkers, loss of therapeutic benefit is associated with relapse of the biomarker. The time course of type 2 responsive biomarker change does not directly track clinical benefit – for example is may change prior to clinical benefit or it may relapse (e.g., when stimulation is turned off) even as clinical benefits persist. Type 2 responsive biomarkers are used in dose instructions under the assumption they are mechanisms leading to clinical benefit – and for this reason type 2 biomarker changes are presumed necessary prior to desired clinical endpoint changes. Theory of mechanisms are revised, leading to a changed biomarker or how the biomarker is analyzed for dose instructions. For example, the mechanism is updated from an increase in local brain activity to a change on brain region connectivity. However, if a new theory does not lead to a change in device or dose instructions, it has no new impact of clinical outcomes.
Predictive biomarkers do not respond to therapy dose but inform dose optimization to clinical endpoints. More specifically, predictive biomarkers are used in dose instructions assuming (or ignoring) if they are directly changed by therapy dose. Predictive biomarkers can be used to personalize neuromodulation: 1) Prior to treatment, in treatment planning; 2) Immediately before treatment during treatment installation or set-up; or 3) during treatment (online). Predictive biomarkers may be measured repeatedly, and dose adjusted iteratively; dose instructions thus prescribe a loop. However, the structure of the loops is unique to the biomarker type (Figure). Thus, assumption about the nature of the biomarker (type) governs implementation of distinct dose instruction loops.
Predictive biomarkers include neuronavigation, play-back type, gated type, and evoked type. Baseline structural imaging used for neuronavigation (e.g., MRI) and/or current flow modeling is a predictive biomarker. Functional imaging is a predictive biomarker so long as dose is not adjusted based on its change to stimulation. Play-back type predictive biomarkers record physiological activity and provide stimulation matching a spatial or temporal aspect of the activity. Evoked type predictive biomarkers, common in neuromodulation, respond to a test stimulation dose that is distinct form the optimized therapy dose.
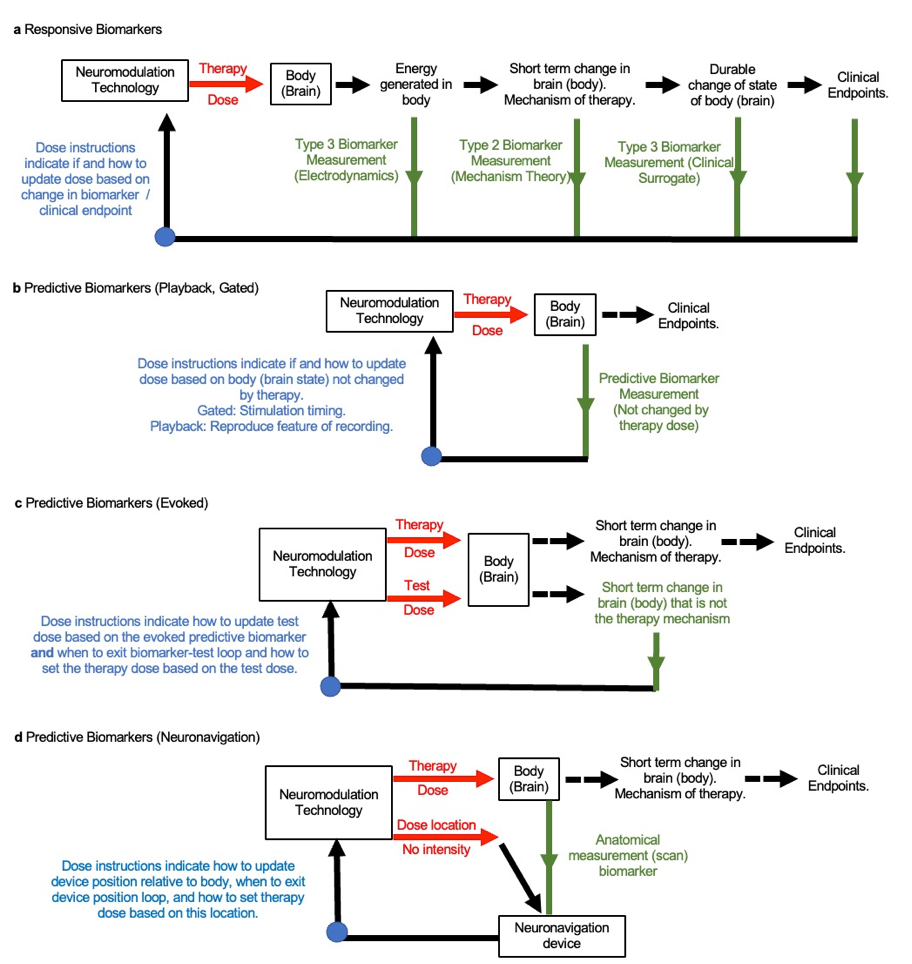
Biomarkers are not exclusive: A therapy dose instruction can use more than one biomarker, but each biomarker needs to be applied in its associated loop structure. The same biomarker may be classified as a different type in distinct therapies (e.g., a discovery causes the role of a biomarker to be revaluated), and this will be associated with different loop structure. The classification here is based only on how the biomarker is used – it is based on empirical practice.
Computational models can be used with any biomarker and are thus serve as tools in dose instructions using the specified biomarker types. Closed-loop systems automate dose instructions using the specified biomarker types. Any measurement made before, during or after stimulation that is not used to adjust dose is not a biomarker per this definition but may enhance background knowledge.
Neuromodulation therapy design is the development of technology and associated dose instructions (loops based on biomarkers). Prior clinical experience and theories on mechanism inspire the design of neuromodulation, but outcomes depend only on dose. The success of a clinical intervention validates the device technology and dose instructions, but does not establish the mechanism (e.g., assumptions about role of biomarker) is correct. Limitations in clinical success inspire new devices (dose possibilities) or dose instructions (loops based on biomarkers). Indeed, like a thermostat and heater regulate room temperature without a complete model of the environment, biomarker and dose instructions can regulate outcomes despite unknowns. Biomarkers, of distinct types, thus underpin the development and implementation of personalized neuromodulation.
Read this next
What Silicon Valley needs to know about the brain, and what it doesn't - Part I
Silicon Valley wants to get inside your head - literally. While these projects adopt mixes of commercial stealth and elaborate publicity, and the breadth of applications spans the entire human condition, all these technologies take one of two product forms: Either as a chip implanted in your head transmitting to a computer or as a wearable cap transmitting to a computer.
Neuromodulation for COVID-19, Neuromodec update 7: Electronic noses, NIH “RECOVER” recruits’ patients, New prospects.
This is the 7th update from Neuromodec (August 5, 2022) on the use of Neuromodulation for COVID-19 as part of Neuromodec’s initiative to educate on and advance treatments.
NYU Langone Hospital Announces At-Home Virtual Brain Stimulation Program
The NYU Langone Health medical system announced at-home service for non-invasive (wearable) neuromodulation...